【tensorflow2.0】学习笔记
🗓 2020年02月08日 📁 文章归类: TensorFlow
版权声明:本文作者是郭飞。转载随意,标明原文链接即可。
原文链接:https://www.guofei.site/2020/02/08/tf2.0.html
基本配置
安装 cuda
GPU支持
先在 nvidia 官网安装 cuda https://developer.nvidia.com/cuda-downloads
然后安装 tensorflow-gpu
pip install tensorflow-gpu
列出可用的设备
tf.config.experimental.list_physical_devices()
tf.config.experimental.list_physical_devices("GPU")
tf.config.experimental.list_physical_devices("CPU")
看 tensor 所用的资源
x = tf.random.uniform([3, 3])
x.device
切换回CPU的方法
import os
os.environ["CUDA_VISIBLE_DEVICES"] = "-1"
运行,并看用了哪个设备
tf.debugging.set_log_device_placement(True) # 每个算子都会显示详细信息
with tf.device('/device:GPU:0'):
a = tf.constant([[1.0, 2.0, 3.0], [4.0, 5.0, 6.0]])
b = tf.constant([[1.0, 2.0], [3.0, 4.0], [5.0, 6.0]])
c = tf.matmul(a, b)
print(c)
主要模块
- tf.data 加载数据
- tf.keras 构建模型(estimator验证模型,hub迁移学习)
- eager mode 运行和调试
- 分发策略做分布式训练
- SavedModel 导出模型
- 部署模型TensorFlow Server, TensorFlow Lite(Android & IOS), TensorFlow.js
Tensor基本操作
生成
tf.zeros([10, 5])
运算
tf.add(1, 2)
tf.add([1, 2], [3, 4])
tf.square(5)
tf.reduce_sum([1, 2, 3])
tf.square(2) + tf.square(3)
矩阵计算
tf.matmul([[1]], [[2, 3]])
map
tf.data.Dataset.from_tensor_slices([1, 2, 3, 4, 5, 6])
ds_tensors.shuffle(2).batch(2).map(tf.square)
# 这是个 <dataset> 用法见于相关内容
还有啥
tf.where
tf.print
自动微分
x = tf.ones((2, 2))
# persistent=True 就可以多次运行 t.gradient,否则只能运行一次
with tf.GradientTape(persistent=True) as t:
t.watch(x)
y = tf.reduce_sum(x)
z = tf.multiply(y, y)
# Derivative of z with respect to the original input tensor x
dz_dx = t.gradient(z, x)
dz_dx
dz_dy = t.gradient(z, y)
dz_dy
高阶微分
x = tf.Variable(1.0) # Create a Tensorflow variable initialized to 1.0
with tf.GradientTape() as t:
with tf.GradientTape() as t2:
y = x * x * x
# Compute the gradient inside the 't' context manager
# which means the gradient computation is differentiable as well.
dy_dx = t2.gradient(y, x)
d2y_dx2 = t.gradient(dy_dx, x)
训练相关
v = tf.Variable(3.0)
v.assign(tf.square(v))
tf.assign_sub # 手写梯度下降时用的多
激活函数
tf.nn.softmax
完整案例
简陋款
import tensorflow as tf
import numpy as np
class MyModel():
def __init__(self):
self.W = tf.Variable(1.0)
self.b = tf.Variable(1.0)
def __call__(self, x):
return self.W * x + self.b
my_model = MyModel()
# %%
n_samples = 1000
X = np.random.rand(n_samples, 1) * 5
y = 5 * X + np.random.randn(n_samples, 1) + 2
import matplotlib.pyplot as plt
plt.plot(X, y, '.', label='true')
plt.plot(X, my_model(X), '.', label='predict')
plt.legend()
plt.show()
# %%
def loss(predict_y, true_y):
return tf.reduce_mean(tf.square(predict_y - true_y))
def train(model, X, y, learning_rate):
with tf.GradientTape() as t:
current_loss = loss(my_model(X), y)
dW, db = t.gradient(current_loss, [my_model.W, my_model.b])
model.W.assign_sub(learning_rate * dW)
model.b.assign_sub(learning_rate * db)
# %%
Ws, bs = [], []
losses = []
for epoch in range(100):
Ws.append(my_model.W.numpy())
bs.append(my_model.b.numpy())
losses.append(loss(my_model(X), y))
train(my_model, X, y, learning_rate=0.01)
# %%
fig, ax = plt.subplots(3, 1)
ax[0].plot(Ws, label='W')
ax[0].plot(bs, label='b')
ax[1].plot(losses, label='loss')
ax[2].plot(X, y, '.', label='true')
ax[2].plot(X, my_model(X), '.', label='predict')
ax[0].legend()
ax[1].legend()
ax[2].legend()
plt.show()
tf.func
类似这样的,但速度更快
@tf.function
def add(a, b):
return a + b
有时候想限定输入值的类型,如果不符合,让它报错(例如,上面这个例子输入数字和字符串都可以)
# 注意,shape也要严格满足,不然也会报错
@tf.function(input_signature=(tf.TensorSpec(shape=[None,None], dtype=tf.int32),))
def next_collatz(x):
print("Tracing with", x)
return tf.where(x % 2 == 0, x // 2, 3 * x + 1)
next_collatz(tf.constant([[1, 2], [3, 4]]))
# 或者用现成的
@tf.function
def double(a):
return a + a
double_string = double.get_concrete_function(tf.TensorSpec(shape=None, dtype=tf.string))
double_string(tf.constant(["a"]))
tf.estimator
tf.estimator
tf.estimator
预装了很多机器学习模型- 继承自
tf.estimator.Estimator
- 可以自定义 estimator: https://www.tensorflow.org/tutorials/estimator/keras_model_to_estimator
需要做这几件事:
- Create one or more input functions.
- Define the model’s feature columns.
- Instantiate an Estimator, specifying the feature columns and various hyperparameters.
- Call one or more methods on the Estimator object, passing the appropriate input function as the source of the data.
input functions
可以自己写一个函数, return features, labels(具体不写了,用的时候查)
更推荐的方法是使用 tf.data
feature columns
tf.feature_column
例如:
my_feature_columns = [
tf.feature_column.numeric_column(key='feature_name1',dtype=tf.float32) # 实数特征
tf.feature_column.categorical_column_with_vocabulary_list(key='feature_name2', vocabulary_list=['A', 'B', 'C', 'D']) # 分类特征
tf.feature_column.crossed_column(['age', 'sex'], hash_bucket_size=100) # 字段之间的交叉特征
]
[NumericColumn(key=’feature1’, shape=(1,), default_value=None, dtype=tf.float32, normalizer_fn=None)]
这个可以很复杂,参见 this guide
Instantiate an estimator
有很多种,例如
tf.estimator.DNNClassifier
for deep models that perform multi-class classification.tf.estimator.DNNLinearCombinedClassifier
for wide & deep models.tf.estimator.LinearClassifier
for classifiers based on linear models.
classifier = tf.estimator.DNNClassifier(
feature_columns=my_feature_columns,
# Two hidden layers of 30 and 10 nodes respectively.
hidden_units=[30, 10],
# The model must choose between 3 classes.
n_classes=3)
# linear classifier (logistic regression model)
tf.estimator.LinearClassifier(feature_columns)
Train
classifier.train(
input_fn=lambda: input_fn(train, train_y, training=True),
steps=5000)
print('\nTest set accuracy: {accuracy:0.3f}\n'.format(**eval_result))
prediction
predictions = classifier.predict(
input_fn=lambda: input_fn(predict_x))
# predictions 是一个 generator
classifier.evaluate(input_fn)
boost tree 的例子
# Since data fits into memory, use entire dataset per layer. It will be faster.
# Above one batch is defined as the entire dataset.
n_batches = 1
est = tf.estimator.BoostedTreesClassifier(feature_columns,
n_batches_per_layer=n_batches)
# The model will stop training once the specified number of trees is built, not
# based on the number of steps.
est.train(train_input_fn, max_steps=100)
# Eval.
result = est.evaluate(eval_input_fn)
# 注:参数
params = {
'n_trees': 50,
'max_depth': 3,
'n_batches_per_layer': 1,
# You must enable center_bias = True to get DFCs. This will force the model to
# make an initial prediction before using any features (e.g. use the mean of
# the training labels for regression or log odds for classification when
# using cross entropy loss).
'center_bias': True
}
tf.data
tf.data.experimental.CsvDataset 用来直接从 gzip中读取csv
下载
TRAIN_DATA_URL = "https://storage.googleapis.com/tf-datasets/titanic/train.csv"
train_file_path = tf.keras.utils.get_file("train.csv", TRAIN_DATA_URL)
# 从网上下载csv,并存到 ~\.keras\datasets 这个文件夹下。
# 返回的 train_file_path 是一个str,内容是存储路径
读取本地csv
dataset = tf.data.experimental.make_csv_dataset(
file_pattern=train_file_path,
batch_size=5, # Artificially small to make examples easier to show.
label_name='survived',
na_value="?",
num_epochs=1,
ignore_errors=True)
dataset.take(1) # 返回的是一个generator
# dataset.take(1) 是一个 generator,里面是一个 batch
for batch, label in dataset.take(1):
for key, value in batch.items():
# 这里的key是 str,内容是 字段名
# value是tf.Tensor,内容是 值(batch_size维)
print(key, value)
关注以下参数
- batch_size
- label_name
- select_columns:读入指定的列,其它列被忽略
- column_defaults
然后,还可以:(代码省略,用的时候查)
- 把字符串格式的值,转为float格式
- dataset.map 后的返回值,可以直接作为
model.fit
的入参
numpy
from sklearn import datasets
X, y = datasets.make_classification(n_samples=1000, n_features=10)
dataset = tf.data.Dataset.from_tensor_slices((X, y))
# dataset 的类型是 <BatchDataset>
dataset = dataset.shuffle(100).batch(64)
使用
# 取一个batch
dataset.take(1)
# 可以直接使用
model.fit(dataset, epochs=10)
model.evaluate(dataset)
numpy 与 tensor 的算子都可以直接相互套用。
而且他们共享内存,但如果用GPU,那么会做一个copy
import numpy as np
ndarray = np.ones([3, 3])
images
import pathlib
data_dir = tf.keras.utils.get_file(origin='https://storage.googleapis.com/download.tensorflow.org/example_images/flower_photos.tgz',
fname='flower_photos', untar=True)
data_dir = pathlib.Path(data_dir)
NLP
需要下载两个包,分别是训练好的模型、数据
!pip install -q tensorflow-hub
!pip install -q tensorflow-datasets
使用训练好的模型
下面是一些训练好的 word embedding 模型
- google/tf2-preview/gnews-swivel-20dim/1
- google/tf2-preview/gnews-swivel-20dim-with-oov/1 与上面这个相同,但是 2.5% 作为OOV,因此适合用来 task 的 vocabulary 不重合的情况。
- google/tf2-preview/nnlm-en-dim50/1 很大的模型,1M vocabulary + 50 dimensions
- google/tf2-preview/nnlm-en-dim128/1 更大的模型 1M vocabulary + 128 dimensions
使用方法有两种:
第一种使用方法
import tensorflow_hub as hub
embed = hub.load("https://hub.tensorflow.google.cn/google/tf2-preview/gnews-swivel-20dim/1")
embeddings = embed(["cat is on the mat", "dog is in the fog"])
第二种使用方法
hub_layer = hub.KerasLayer("https://hub.tensorflow.google.cn/google/tf2-preview/gnews-swivel-20dim/1", output_shape=[20],
input_shape=[], dtype=tf.string)
model = keras.Sequential()
model.add(hub_layer)
model.add(keras.layers.Dense(16, activation='relu'))
model.add(keras.layers.Dense(1, activation='sigmoid'))
model.summary()
完整的代码
导入包
import tensorflow as tf
!pip install -q tensorflow-hub
!pip install -q tensorflow-datasets
import tensorflow_hub as hub
import tensorflow_datasets as tfds
print("Version: ", tf.__version__)
print("Eager mode: ", tf.executing_eagerly())
print("Hub version: ", hub.__version__)
print("GPU is", "available" if tf.config.experimental.list_physical_devices("GPU") else "NOT AVAILABLE")
train_validation_split = tfds.Split.TRAIN.subsplit([6, 4])
(train_data, validation_data), test_data = tfds.load(
name="imdb_reviews",
split=(train_validation_split, tfds.Split.TEST),
as_supervised=True)
train_examples_batch, train_labels_batch = next(iter(train_data.batch(10)))
train_examples_batch
下载已经训练好的模型,作为 embedding
embedding = "https://hub.tensorflow.google.cn/google/tf2-preview/gnews-swivel-20dim/1"
hub_layer = hub.KerasLayer(embedding, input_shape=[],
dtype=tf.string, trainable=True)
hub_layer(train_examples_batch[:3])
用 keras 建模
model = tf.keras.Sequential()
model.add(hub_layer)
model.add(tf.keras.layers.Dense(16, activation='relu'))
model.add(tf.keras.layers.Dense(1, activation='sigmoid'))
model.summary()
标准流程了
model.compile(optimizer='adam',
loss='binary_crossentropy',
metrics=['accuracy'])
history = model.fit(train_data.shuffle(10000).batch(512),
epochs=20,
validation_data=validation_data.batch(512),
verbose=1)
加上一个模型评估
results = model.evaluate(test_data.batch(512), verbose=2)
for name, value in zip(model.metrics_names, results):
print("%s: %.3f" % (name, value))
with tf.Graph().as_default():
pass
slim
很有用的东西,可以不用设置中间变量,给函数赋值
slim=tf.contrib.slim
with slim.arg_scope([slim.conv2d,slim.fully_connected],weights_regularizer=slim.l2_regularizer(weights_decay)):
pass
队列和同步运算
Enqueue,Deq ueue,MutexAcquire,MuterRelease
控制流
Merge,Switch,Enter,Leave,NextIteration
x=tf.placeholder(shape=(None,6),dtype=tf.float32)
w1=tf.Variable(tf.random_normal(shape=(6,2)))
a=tf.matmul(x,w1) #矩阵乘法
sess.run(tf.global_variables_initializer())
sess.run(a,feed_dict={x:rv.rvs(size=(3,6))})
有很多已经训练好的模型,可以直接下载下来作为预训练模型
https://github.com/tensorflow/models
keras
人脸检测
人脸检测:检测并定位图片中的人脸
人脸关键点检测:返回五官和轮廓关键点的位置
人脸属性检测:年龄、种族、性别、情绪
移动端
两种方法加快运行速度
1. 量化
quantitative
例如,float32变成int8
训练时不能量化,因为牵涉到梯度。
部署时可以量化,因为神经网络对噪声的鲁棒性很强
性能指标
1. 人脸识别
Top k 错误率
Top k 召回率
识别速度
注册速度(注册一个人的时间)
2. 聊天机器人
- 回答与问应当语义一致、语法正确、逻辑正确
- 有趣、多样,少一些安全回答(好啊,是啊之类的)
- 个性表达一致
其它
tf.train.batch
tf.train.shuffle_batch
lstm:dropout
其它2
autoencoder的代码还没有review
tensorboard
tf.summay.scalar # 标量数据,如准确率,损失值
tf.summay.histogram # 参数数据,如weights,bias
tf.summay.image # 图像数据
tf.summay.audio # 音频
tf.summay.FileWriter # 计算图结构
RNN
- 听:语音识别(Speech To Text),从音频到文本
- 说:语音合成(Text To Speech),从文本到音频
- 读:文本理解(Text Understanding),从文本到语义
- 写:文本生成(Text Generation),从语义到文本
- 文本理解
- 新闻自动分类(文本分类)
- 用户评论分析(情感分析)
- 文本生成
- 翻译
图像识别中,图片大小的问题可以通过裁剪、缩放解决,但文本处理中并不能。
对话机器人
- 基于规则:人工标注,对语料进行数据挖掘,来减少人工标注的工作量
- 基于检索
- 基于生成模型
您的支持将鼓励我继续创作!
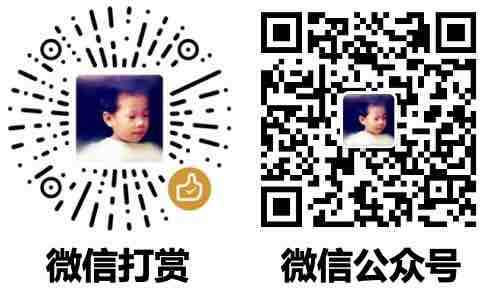